Top 4 Must-Read Speech Data Papers
Since 2017, industry experts have been claiming that data has usurped oil’s position as the world’s most valuable resource. Such is the hype around data, that, in 2012, the data science profession was even declared the sexiest job of the 21st century.
Speech technologies are one of the main Artificial Intelligence drivers and are still exploding the market, as evidenced by insights from Fortune Business Insights. However, when browsing top speech conferences like this past year’s INTERSPEECH 2020, you may not easily find speech data research, so I picked out 4 of the top data papers published and discuss them in this article.
1. “MLS: a Large-Scale Multilingual Dataset for Speech Research”, by Facebook.
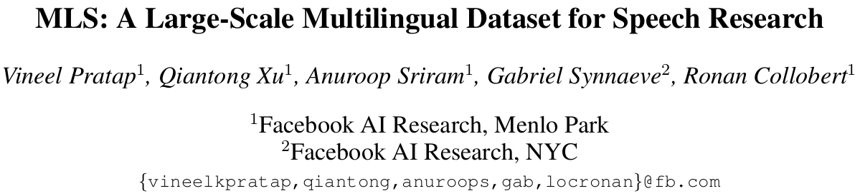
The LibreSpeech dataset has been one of the best-known datasets for Automatic Speech Recognition (ASR) development, being one of the fist large-scale 1000-hour corpora since the era of deep learning products began. The drawback is that it is an English-only dataset, so it has limited use for researchers working on other languages. Facebook has answered the call for the extensibility of this corpus to other languages by increasing their LibreSpeech style corpora by hundreds to thousands of hours of data for most of the 8 languages as seen in Table 1.
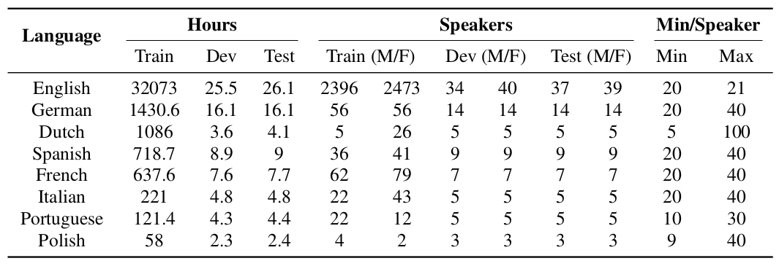
Table 1 – MLS Corpus Statistics
This dataset will open the possibilities for new research in ASR and TTS as the original LibreSpeech dataset did for English.
2. DiPCo – “Dinner Party Corpus”, by Amazon
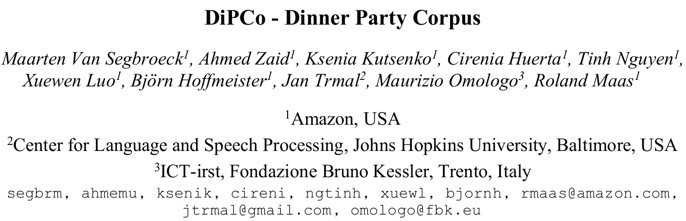
The Dinner Party problem is a NextGen virtual assistant issue which still has a long road ahead but great market potential. These days, virtual assistants are dominating tasks like acting as the kitchen timer or setting reminders, and now are moving into much more versatile functions like placing online orders or controlling home appliances. However when users are far away from the device and/or multiple people are talking at the same time, often with music or noise in the background, results can suffer, and the expectations of the user also decline.
The truth is this area is growing because we find our assistants useful, and we want more from them. In their paper, Amazon released a development/test set for researchers to use. The dataset contains 5.33 hours of speech from 10 dinner party scenarios with four Amazon employees in each and a setup as pictured in Figure 1. Its is reasonably gender balanced (19/13 M/F). each person is recorded by 1 close-up mic and 5 seven-channel far-field mics, yielding 39 mic channels.
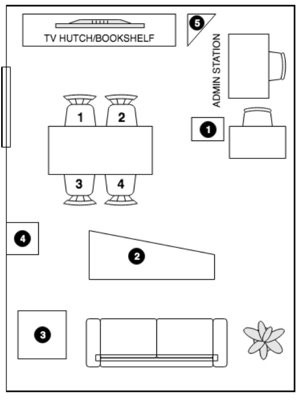
Figure 1.
3. “Developing an Open-Source Corpus of Yoruba Speech” by Google
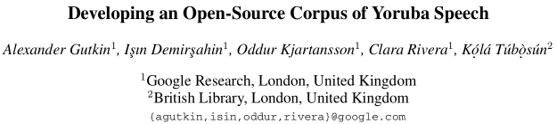
Low-resource languages are often the bane of speech technology expansion. Even for English, many use cases are still difficult, and in the case of most languages, almost no resources exist. This slows down research and development and increases the cost for work in these languages. In theirs recente work, Google researchers have released a 4 hour dataset with 36 speakers. The corpus was recorded at 48kHz, manually checked and designed for work in speech synthesis or TTS (Test-to-Speech). ir could also be used for tasks like ASR or Speaker adaptation as well as expanding multilingual speech models where speakers from the 22 million-strong Yoruba speaker population would be helpful.
Number 4: “Data balancing for boosting performance of low-frequency classes in SLU” by Amazon
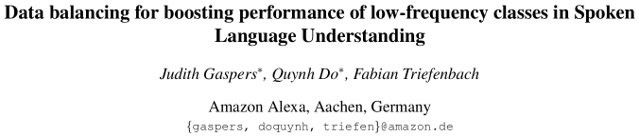
Spoken Language Understanding (SLU) has emerged as a new era of research with the popularization of virtual assistant where speech recognition and natural language understanding applications meet in the middle. For applications which focus on multiple language, is it no surprise once again that languages other than English suffer from a lack of resources. In the Amazon work, experiments are done with machine translated data to boost low-frequently classes, while maintaining the strong performance on the long tail of low-frequency classes, while maintaining the strong performance of head classes which do not have insufficient data. Key findings included the point that where some real data was available, the machine translated data hurt performance but in cases of extremely deficient classes it was helpful.